Automated Oracles
Early warning systems buy time and provide a basis for prompt action. They use data to gain insights into potential future scenarios. But they run up against limits, especially in connection with pandemics.
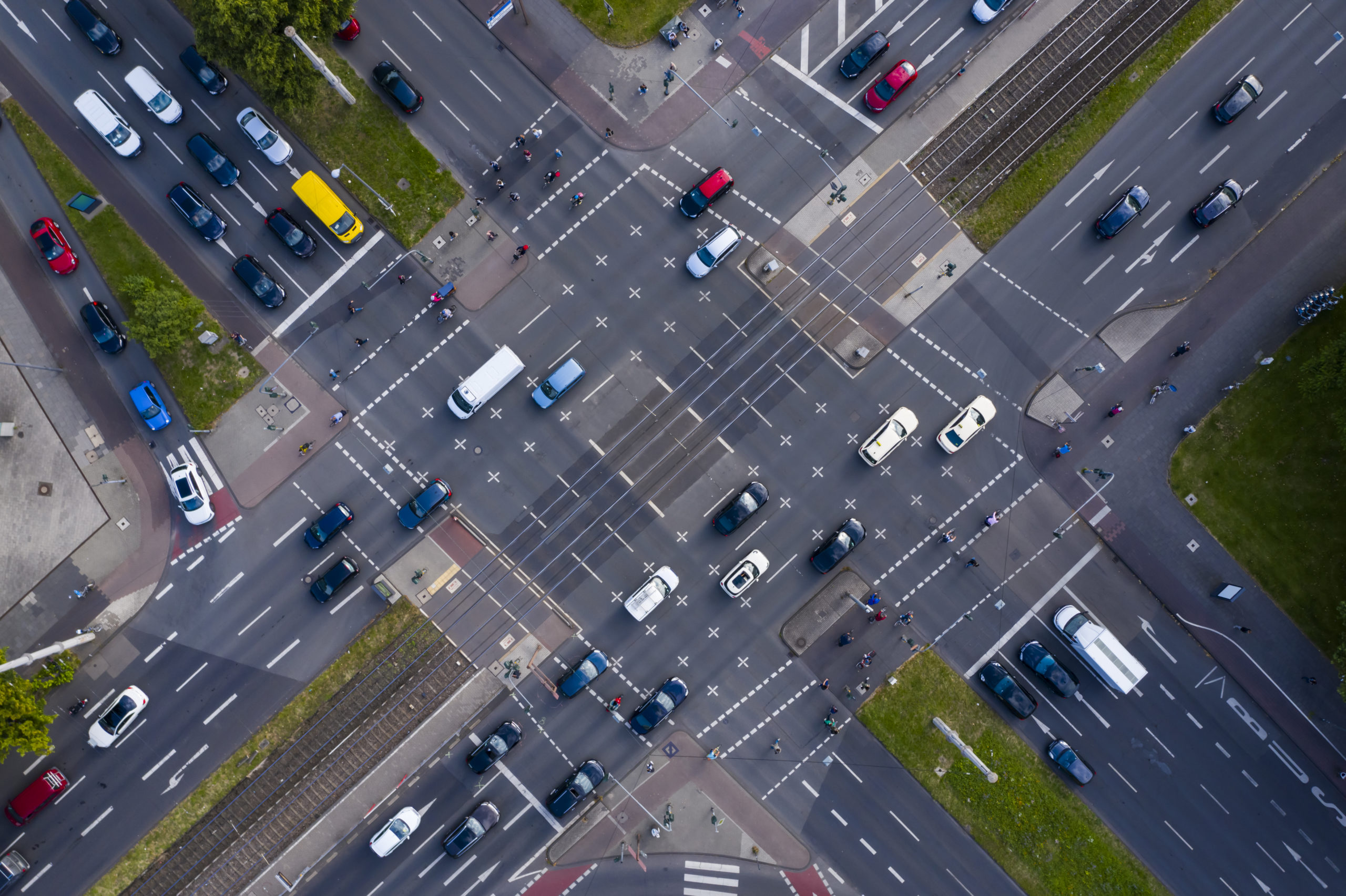
Preparation is the buzzword for efficient action. If you know about a traffic jam, you can drive around it, when a drought is imminent, you can save water. The coronavirus revealed a world unprepared. By mid-2020, more than half a million people had died from Covid-19 infection. And the question arises: Why is there no early warning system for pandemics?
Early warning systems are already standard in a number of other contexts. On December 26, 2004, the ocean floor off the coast of northern Sumatra shifted. This underwater earthquake was one of the strongest in a century, measuring 9.3 on the Richter scale. Its tremors caused waves to surge and slam ever higher against the island and many other coastlines of the Indian Ocean. Nobody was prepared, and more than 25,000 people died. A consortium led by the German Research Centre for Geosciences (GFZ) in Potsdam then set up an early warning system in the Indian Ocean. A network of seismometers detects earthquake epicenters, and satellites measure movements of the earth’s surface via GPS. If the system were to respond to every vibration, there would be many false alarms. GPS buoys and pressure sensors on the ocean floor help measure each wave following a quake. The data are transmitted to a processing center and compared with previous records. This wealth of data yields models that can inform operators within minutes of the speed, direction, and magnitude of potential tsunamis—in other words, whether they are harmless or hazardous.
Analysis via machine learning
Early warning systems can also help companies. One example lets them prepare for disruptions in their supply chains. Resilience360 Supply Watch is the name of a system used by the German logistics company DHL. The program defines around 140 risk categories, including financial, environmental, and social factors. Are media outlets reporting criminal activity in a certain region? What is the incidence rate of quality complaints? How do inventory levels look—do warehouses have sufficient stock? The DHL system analyzes data from up to 30 million online and social media posts and makes the results of its risk analyses available to clients. The program itself continuously evaluates the relevance and potential consequences of its data. It can do this with the help of machine learning.
Machine learning essentially means that a system automatically compiles new information and evaluates it in relation to what it already knows. For example, a commuter might normally need twenty minutes to drive from A to B. But the route now has a construction site. Because other drivers have already had to wait, an app can inform the commuter before leaving home that today’s drive will take an additional thirty minutes. The system analyzes thousands of construction sites in this manner. Based on the overall pool of data it collects, at some point it might determine that whenever an accident blocks one lane of a particular route, the drive will be delayed by approximately forty minutes. Then the app can report this disruption immediately, without waiting to analyze the results from the first drivers to complete the route.
If we make perfect use of a road’s capacity, or have the maximum number of cars complete a route within an hour, that is an unstable system.
Effectiveness in numbers
Early warning systems often draw on millions or even billions of data sets. Depending on the field in question, that might be anything: from historical records to tree rings to Twitter posts. Machine learning helps organize these files. It models the interplay of different factors.
Dirk Helbing, a professor of computational social science at ETH Zurich, studies how analyzing large volumes of data can help us do things like improve the flow of traffic. The goal is for systems to be stable. “If we make perfect use of a road’s capacity, or have the maximum number of cars complete a route within an hour, that is an unstable system,” he says. “The flow will break down. The road’s capacity will drop, and the result will be congestion.” It is better for the road to be used at a constant rate, but not at its maximum capacity. This approach makes the system less susceptible to disruption. “Every access road or exit, every lane change, every acceleration or brake action—all of these are potential disruptions.” Machine learning can be used to analyze these disruptive factors and derive a collective picture of driving behavior. “It won’t do you any good to furnish just one car with a program,” says Helbing. Efficiency comes from a swarm structure—namely, when many cardrivers make their driving patterns available for data analysis. “Analyzing about 40 percent of the cars could significantly improve traffic flow.”
A single post on social media is of no use for a projection. But if fever posts cluster in a certain location, that can be an indication.
Social media as a warning system
Data scientist Dr. Avaré Stewart also used swarm intelligence when she led the M‑Eco project at Leibniz University Hannover. Her team wanted to analyze data from social media to develop an early warning system for pandemics. “People often post about things like fever symptoms or visits to the doctor,” says Stewart. “A single post is of no use for a projection. But if fever posts cluster in a certain location, that can be an indication.”
The advantage of social media lies in the immediate nature of the data, which can help flag an outbreak early on. But it is difficult to establish a solid basis for these data. “People post about their dog, their niece, or their next vacation. Things that don’t necessarily have anything to do with illness. To increase the quality of your data, you have to filter it.” If the filter is too fine, you will lose important information. If it’s too coarse, the system is unusable. “Finding the right balance here is the biggest challenge,” says Stewart.
Another problem lies in determining how early a warning should be. “We’re comparing the present with knowledge about the past,” says Stewart. “So we can only issue a warning when something has already happened.” In the case of a pandemic, that means when people are already infected. “For us, it was too early to predict potential pandemics solely on the basis of our data.” That was a factor in discontinuing the project when its EU funding ran out.
Coronavirus alert app: Developed too slowly?
Germany’s coronavirus alert app is an early warning system as well. It doesn’t actually prevent infections, but does help to slow their spread. Now that a number of problems in connection with this complex project have been solved, the app is more reliable at generating new numerical codes every few minutes, which it sends to all nearby smartphones via Bluetooth. Unlike such apps in other countries, no centralized computer knows which phones have been in contact with other devices. The codes are only saved on the smartphones—which cannot identify any other users. Google and Apple, the two largest providers of smartphone operating systems, have access to all the codes and can link them to the respective devices. If a user registers a positive test result, this information is sent to the two IT giants, which then send alerts to all smartphones whose users have contacted or been in close spatial proximity to the infected individual.
Because Germany does not collect data in a centralized location or track GPS signals and because it guarantees anonymity, it needed more time to develop its app than other countries such as China, whose tech giant Alibaba took only three days. However, it is unclear how well the app worked in China during the first few weeks. An investigation by the Berlin-based Mercator Institute for China Studies reports more than 50,000 complaints of technical glitches in the first week of app usage alone.
Extensive debate about data privacy before the app was introduced in Germany seems to have had a favorable effect on its acceptance in the country. The number of users is a crucial factor in how well this system developed by SAP and Telekom can function. In the week following its release, the coronavirus alert app was downloaded by around 15 percent of the German population. In Austria, a similar app called Stopp Corona was available a month earlier, but was only being used by around 8 percent of the population three months after its release. Austria has since improved its warning app and guaranteed the same level of data privacy as in Germany.
Safety versus speed
In Germany, every infection reported on the app is verified. People who register a positive test result have to provide confirmation. This eliminates the possibility of false alarms. However, it also takes more time for alerts to be sent. And with every minute that goes by, the risk of infecting more people increases. What is more important, safety or speed? This dilemma keeps appearing in conjunction with early warning systems. And even the best systems cannot provide certainty about the future, but instead only show options. By laying the foundations for knowledge early on, they buy time. That allows people to prepare, and to take precautions sufficiently early to influence the future. The coronavirus app can give warnings. But the users themselves then need to decide to limit their contact with others.
Early warning systems in nature, science, and business
- Elephants have pressure sensors on the soles of their feet that can detect infrasound—around sixteen to twenty hertz below the range of human perception. That enables them to respond early to earthquakes and tsunamis.
- The German-Indonesian Tsunami Early Warning System for the Indian Ocean operated from Jakarta identifies earthquake epicenters within twenty kilometers and calculates their magnitude within three or four minutes after occurrence.
- The University of Constance developed its CoronaVis early warning system for intensive-care capacity limits. Its aim is to enable patient transfers before capacities are reached—including to other regions and states within Germany.
- The Ifo institute compiles a business climate index for Germany by surveying around 9,000 companies monthly about their current situation and expectations for the next six months. Investment advisors use the index as an early warning system.